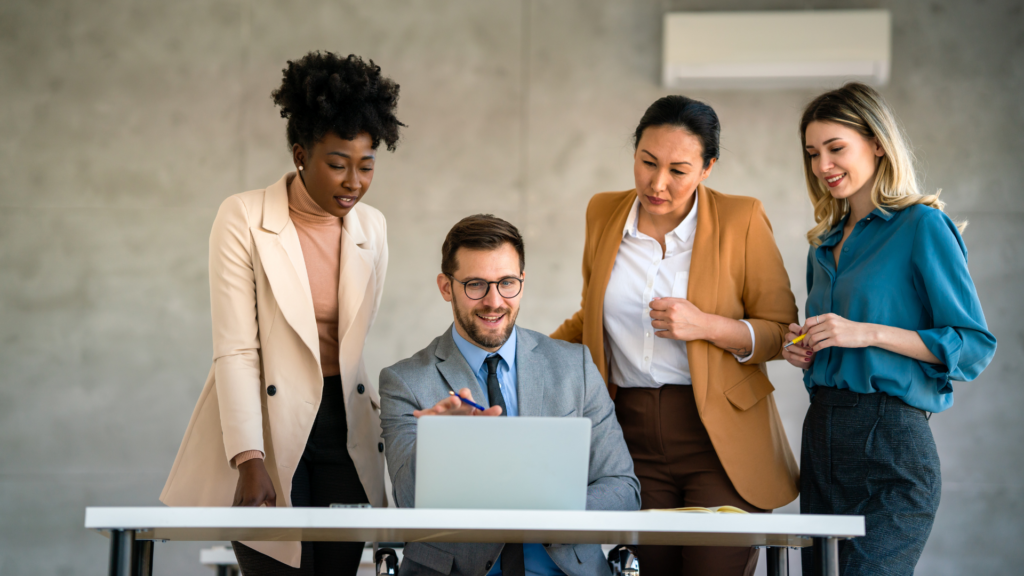
Why Your HR Strategy Needs Machine Learning Now
The human resources landscape is undergoing a seismic shift. Traditional HR practices—reliant on manual processes, gut instincts, and reactive strategies—are struggling to keep pace with the demands of modern workplaces. Enter machine learning (ML), a transformative force poised to redefine how organizations attract, retain, and empower talent. If your HR strategy hasn’t yet embraced ML, you’re not just missing out on efficiency; you’re risking obsolescence in a world where data-driven decisions separate industry leaders from laggards.
The Limitations of Traditional HR Strategies
For decades, HR departments have operated with tools and frameworks designed for a simpler era. Recruitment relied on sifting through stacks of resumes. Employee engagement was measured through annual surveys. Retention strategies were reactive, often implemented only after a star employee handed in their notice. These methods are time-consuming, error-prone, and increasingly ineffective in a world where speed, precision, and personalization are non-negotiable.
Consider the hiring process: a single job posting can attract hundreds of applicants. Manual screening is not only tedious but also riddled with unconscious biases. Even the most well-intentioned HR teams might overlook qualified candidates due to human error or subjective judgment. Similarly, predicting turnover or identifying skill gaps often feels like guesswork without robust data analysis.
This is where machine learning steps in. By leveraging vast datasets and pattern recognition, ML algorithms automate and optimize HR workflows, delivering insights that humans alone cannot replicate.
What Machine Learning Brings to HR
Machine learning is a subset of artificial intelligence (AI) that enables systems to learn from historical data, identify patterns, and make decisions with minimal human intervention. In HR, this translates to:
•
Predictive Analytics: Forecasting turnover, identifying flight risks, and predicting hiring needs.
•
Bias Reduction: Eliminating subjective biases in recruitment and promotions.
•
Personalization: Tailoring employee experiences, from onboarding to career development.
•
Efficiency: Automating repetitive tasks like resume screening and payroll processing.
For example, ML-powered tools can analyze employee behavior, performance metrics, and engagement data to predict which team members are likely to leave. HR can then proactively address concerns—whether through mentorship, flexible work arrangements, or career pathing—before it’s too late.
Key Areas Where ML Transforms HR
1. Smarter Recruitment
Machine learning algorithms excel at parsing resumes, cover letters, and even social media profiles to identify candidates who align with your company’s culture and technical requirements. Tools like AI-driven applicant tracking systems (ATS) rank applicants based on skills, experience, and potential fit, reducing time-to-hire by up to 50%.
But ML goes beyond screening. It can also analyze historical hiring data to identify which sourcing channels yield the best talent, which interview questions predict success, and even which job descriptions attract diverse candidates.
2. Enhanced Employee Engagement
Annual engagement surveys are outdated the moment they’re completed. ML enables real-time sentiment analysis by mining data from communication platforms (e.g., Slack, Microsoft Teams), email interactions, and performance reviews. Natural language processing (NLP) can detect subtle signs of dissatisfaction, such as changes in communication tone or frequency, allowing HR to intervene early.
3. Personalized Learning & Development
Generic training programs often fail to address individual employee needs. ML algorithms analyze skill gaps, learning preferences, and career goals to recommend tailored development plans. For instance, an employee eyeing a leadership role might receive curated courses on conflict resolution, while a technical specialist could get certifications in emerging technologies.
4. Retention Through Predictive Insights
Replacing an employee costs 6–9 months of their salary. ML mitigates this by predicting turnover risks. By analyzing variables like promotion history, workload, peer feedback, and even commute time, algorithms flag at-risk employees, enabling HR to deploy retention strategies proactively.
5. Bias-Free Decision Making
Humans are inherently biased, whether in performance reviews or promotions. ML models, trained on anonymized data, ensure decisions are based on merit. For example, tools like GapJumpers blind hiring managers to demographic details, focusing solely on skills assessments.
Overcoming Implementation Challenges
Adopting ML isn’t without hurdles. Data quality is critical—garbage in, garbage out. HR teams must ensure datasets are clean, diverse, and ethically sourced. Change management is equally vital; employees may fear job displacement or distrust AI-driven decisions. Transparent communication about ML’s role as a tool—not a replacement—is key.
Start small. Pilot ML in one area, like recruitment or engagement analytics, before scaling. Partner with vendors who prioritize ethical AI and explainability, ensuring algorithms aren’t “black boxes.”
The Future of HR is Machine-Powered
Organizations that delay ML adoption will soon find themselves outpaced by competitors who leverage data to build agile, future-ready workforces. Machine learning isn’t about replacing HR professionals—it’s about empowering them to focus on strategic, human-centric tasks while algorithms handle the grunt work.
From reducing bias to predicting turnover, the benefits are too significant to ignore. The question isn’t whether your HR strategy needs machine learning—it’s how quickly you can integrate it.